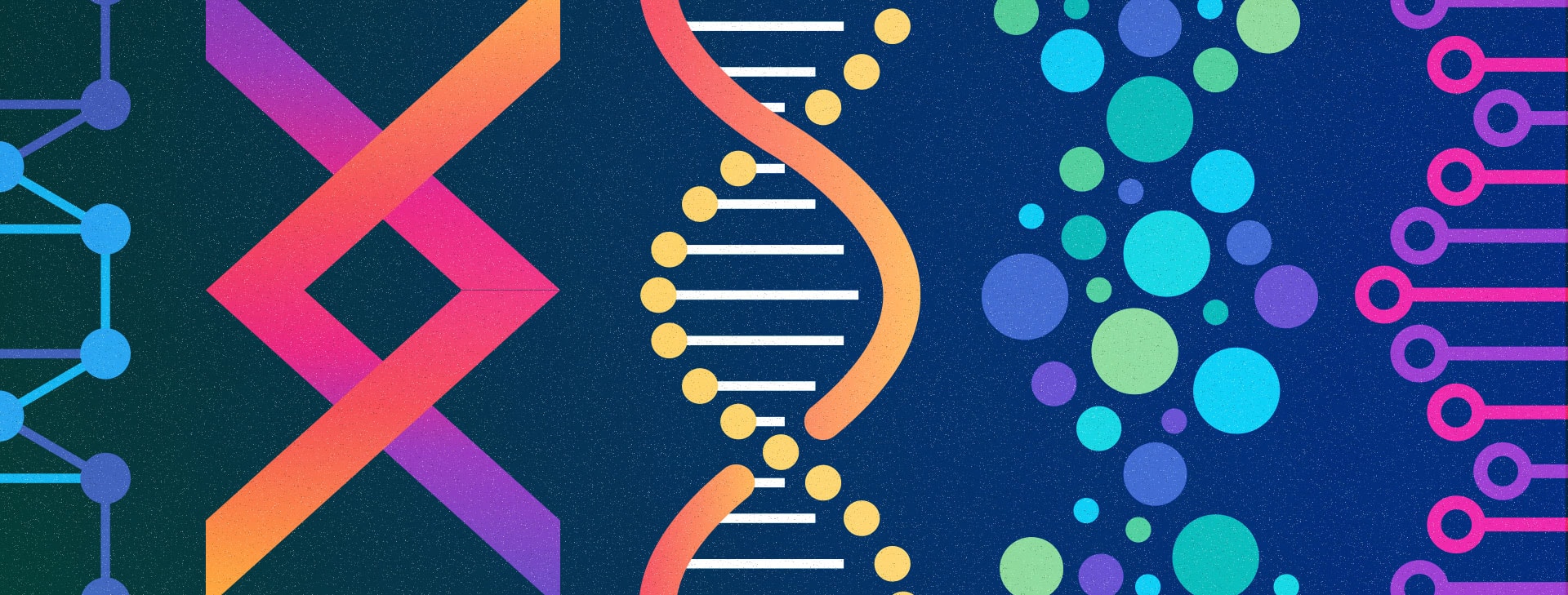
Recommendations to Support the Validity of Claims in NGSS Assessment (Part 2)
A New Framework for Organizing and Evaluating Claims
This is the second in a series of posts by our 2020 summer interns and their mentors based on their project and the assessment and accountability issues they addressed this summer. Sandy Student, from the University of Colorado Boulder, and Brian Gong get things started with a two-part series describing their work analyzing the validity arguments for states’ large-scale Next Generation Science Standards (NGSS) assessments.
In Part 1, we described the challenge of designing large-scale assessments to measure a set of standards as complex and broad as the Next Generation Science Standards (NGSS). We offered recommendations on test design to better align states’ large-scale summative science assessments with the claims implicit in the NGSS and what the state wants to make about student performance. We do not believe, however, that test design alone is adequate to meet this challenge. It is also necessary to reconsider the way that we think about student-level claims based on performance on these assessments.
Goldilocks Claims: Not too Broad, Not too Narrow
It is easy to make claims about students in Achievement Level Descriptors (ALDs) or score reports that are too broad for a test to possibly support them empirically. Broad claims that assert a student’s ability to apply science and engineering practices and crosscutting concepts in each reporting area, for example, inevitably require more evidence than one could acquire from a two-hour test administered every third year of a student’s education. Conversely, the claims that current tests most directly support can seem disjointed and confusing to stakeholders. Descriptions of a student’s abilities relative to the specific Performance Expectations (PEs) that they saw on their test form, for example, could be difficult to interpret without the context of a broader construct to tie those descriptions together.
States must balance the ability to argue for their claims’ validity with the need to maintain useful and comprehensible claims for their original purpose. This leads to our recommendation for states to consider a framework of tiered claims for large-scale assessments.
Tiered Claims to Support Validity and Provide Clarity to Stakeholders
To improve upon current claims and resolve many of the issues discussed here, we propose the use of tiered claims. The defining characteristic of these claims is that unlike the claims about students currently used in ALDs and reporting (Office of Superintendent of Public Instruction, 2020; Science Assessment Team, Office of Superintendent of Public Instruction, 2018), they differentiate between the claims for which there is direct evidence and the claims that are expected to be true but for which the test does not provide direct evidence. Ultimately, the goal of this approach to claims is to provide greater clarity about the evidence available for different claims and to avoid the problems that can result from overly broad claims without making claims so narrow as to lose their meaning.
This claim structure makes it possible to support claims with a relatively similar test to that which is already in operation in Washington, as well as to demonstrate alignment between the test itself and the claims it is intended to support. This is possible because tiered claims are built around recognizing that not all claims are the same: some come directly from the test, while others–perhaps most–require evidence beyond what any one test form can provide.
Creating a Set of Tiered Claims
We propose one approach to creating tiers among claims. In this approach, tiers align with steps in an interpretive validity argument (Kane, 2006): scoring, generalization, extrapolation–see Table 1 for more details on this framework.
Tier I: Scoring and observed performance. These are claims for which the test that a specific student took provides direct evidence. For student-level reporting, phrasing is along the lines of, “[The student] demonstrated the ability to…” followed by a list of specific descriptions of the Disciplinary Core Ideas (DCIs), Science and Engineering Practices (SEPs), and Crosscutting Concepts (CCCs) to which the items on the test form were aligned.
Tier II: Generalization. These are claims for which the test did not provide direct evidence for a given student, but which are defensible given the student’s performance. These are based on generalization from the student’s observed performance to their hypothesized performance on other items they could have seen on the test. This generalization would be based on the list of PEs assessed across all forms, the items available in the item bank, the content of instruction, and other sources of information; these will differ from one state to the next. For student-level reporting, the phrasing would be similar to, “Based on [the student’s] performance, it is probable that he/she/they is/are also able to…” followed by a list of specific descriptions of the DCI/SEP/CCC combinations that the student might have seen, but did not.
Because of the well-documented problem of excessive task/occasion-specific variance in science performance assessment, it is important to distinguish these claims from those for which direct evidence is available.
Tier III: Extrapolation and Trait implications. This tier houses all broader claims about students’ skills, knowledge, abilities and/or futures. Phrasing at this level should read similarly to, “Based on [the student’s] performance on this test, we expect that they are also able to….” In addition to broad claims about student ability, claims about students’ futures that in principle could be investigated empirically, such as associations between current test performance and preparedness for future study, also belong in this tier. This is also the place to locate claims that attempt to draw inferences to the NGSS or even the original Framework on which they are based. These broad inferences are typically impossible to justify with direct evidence from the test and therefore require extrapolating beyond the generalization level in Tier II.
Table 1. Steps of Kane’s validity argument for trait interpretation |
Step | From | To | Evidence Types |
Scoring | Raw test responses | Observed scale score | Expert input to scoring criteria, appropriateness/fit of scoring procedures, interrater reliability, analysis of equating. |
Generalization | Observed scale score | Universe of generalization score | Reliability analysis, generalizability analysis, analysis of the consistency of measurement procedure. |
Extrapolation | Universe score | Target domain score | Analytic: relation of universe of generalization to target domain, think-aloud studies of cognitive processes, analysis of construct-irrelevant variance. Empirical: criterion studies, validity generalization studies, correlations between different measures of the same claimed trait. |
Implication | Target score | Trait implication | Analytic: alignment between target domain and hypothesized structure of trait. Empirical: comparison of hypothesized and apparent relations between different traits. |
It is important to note that within each tier, the quality of evidence can still vary. For example, poor alignment to standards would imply that even at Tier I, the evidence is weak. Conversely, a strong research program linking test performance to future outcomes, would be an instance of strong evidence in Tier III. The strength of evidence for generalizations in Tiers II and II requires particular attention. At Tier II, concerns about generalization stem from prior studies demonstrating large task/occasion-specific variance in science performance assessments (Brennan & Johnson, 2005; Gao et al., 1994; Ruiz-Primo & Shavelson, 1996). For Tier III, we note that “more generalizations and stronger models require more evidence” (Kane, 2013, p. 36); the broader claims made here, for which evidence is currently weakest, require the strongest evidence of all.
Additionally, validation does not end at extrapolation; the consequences and uses of test scores are also a crucial component of validity. This claim framework does not consider the consequences or uses of test scores because our concern is primarily interpretation. It is difficult to consider the validity of actions based on NGSS assessment claims if the claims themselves lack support.
While our work so far has focused on science assessment, this framework could certainly be applied to other large, complex domains. Nearly all state assessments sample from a large body of standards to create test forms, generalize from these forms to the standards as a whole, and use this generalization to support claims about students’ preparation for future study. This means that across disciplines, the framework of tiered claims can provide greater clarity about the relationship between large-scale tests and the claims they are able to support.
We believe that each of the recommendations in this post and in Part 1, implemented on their own, would make it likelier that a state’s claims about their students are defensible. Implemented together, they can form the basis of a stronger validity argument than any that can be constructed for states’ NGSS science assessments today.