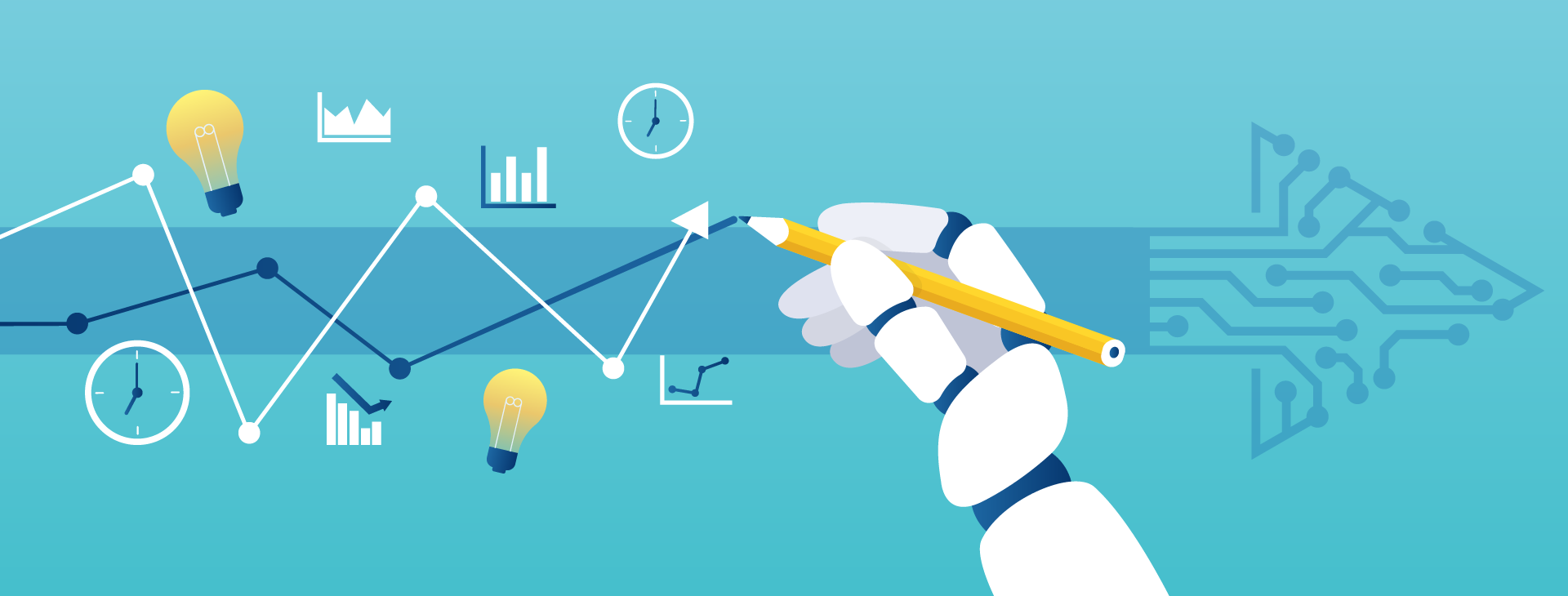
The Future of Assessment in the Age of Artificial Intelligence
The Debut of Gemini Forecasts the Disruption of Testing
For those of us working in disciplinary areas of educational assessment who are afraid of change, I am afraid that the world at large has some bad news: large language models and other forms of generative artificial intelligence (AI) will introduce unprecedented disruption to our work.
I see this as a decidedly positive development, since it forces us to narrow the chasm between clinging stubbornly to more traditional practices of doing business and leaning into technological advances to transform the way we work. At some point, potential bridges that could traverse this chasm simply cannot be built anymore.
A few weeks ago, Google rolled out extended demos and a technical report for its latest engine, appropriately titled Gemini. Its development is an incredible team effort, as showcased by the pages of contributors with vast financial resources dedicated to it. The demos and technical report showcased the mind-blowing capabilities of the first version of this engine. Embedded in Google’s release are myriad examples of this complex model solving problems in core disciplinary areas such as STEM, language arts, and mathematics.
My colleague Will Lorié and I recently wrote a blog post in which we mused about some of the implications of AI for educational assessment in the future. I want to revisit some of these ideas here in light of what Gemini is already capable of doing (and will be able to do even better in the near future). The ideas here reflect not a comprehensive list, but a provocation to think about what I believe are some unavoidable directions of strategic work that will now be increasingly within reach for many organizations.
AI Will Transform Standardized Assessment Life Cycles
I really like the adapted spiral model of assessment development that I came across in Georgia’s original innovative-assessment application, which showcases nicely the iterative nature of this work; I’ve reproduced it here.
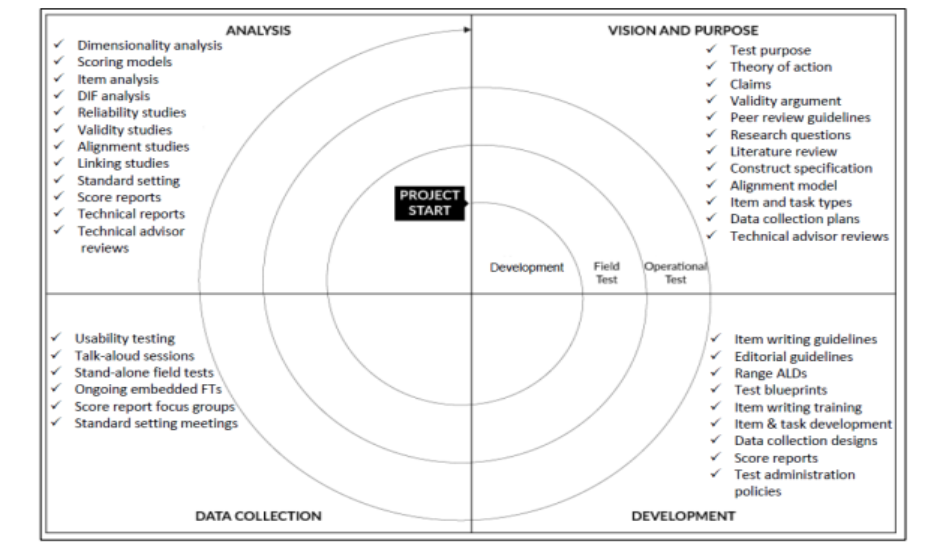
Many of us who have worked for or with professional vendors will know, however, that the implementation of such an assessment development process is often rather slow, costly, and clunky. Put differently, many practical aspects of this work simply feel dated, overly technocratic, fragmented, and relatively unresponsive to the agile needs of assessment users.
AI generally, and generative AI more specifically, have started to reshape the assessment development process, and one can easily envision these systems being overlaid onto many of the component processes in the spiral model. This can speed up the spiral, transforming it from the shell of a snail to a spinning wheel.
For example, AI will likely be able to do the following, among other things, reasonably well:
Vision and Purpose
- Generate alternative representations of theories of action to facilitate critical discussion
- Generate outlines of data collection plans with associated task breakdowns
- Generate syntheses of evidence for technical advisory reviews from a multimodal database
Development
- Generate sample assessment blueprints based on goal and context descriptions
- Generate instances of individual items based on design considerations
- Suggest revisions to items and tasks
- Generate variants of items from item families that share common design features
- Create translated versions of items into many languages
Data Collection
- Generate sample responses for the items, especially open-ended tasks
- Assemble more traditional test forms and collections of tasks for delivery
- Generate automated multimodal feedback and reports based on learner performance
- Adjust delivery aspects to accommodate learners with different needs
Analysis
- Automatically evaluate a wide range of work products for a wide range of competencies
- Automatically analyze multimodal log files for evidence of metacognitive processes
- Automatically evaluate model-data fit for common scaling models
- Suggest revisions to item and task specifications based on data analyses
Humans in the loop will then have a changing role: as designers of specifications for interactions with generative AI algorithms and as critical consumers of their output, rather than as the sole generators and reviewers of output. Luckily, there is a growing need for humans in the loop for both AI generation and AI applications.
AI Will Shift The Foci of Teaching, Learning, and Assessment
- Teaching and Learning Transformations
What do AI-based developments mean for the pedagogical work of teaching and learning in the K-12 space? Put simply, innovation initiatives in learning that leverage digital technologies deserve strong support. A recent blog on generative AI described the promise of these technologies more broadly for teachers in particular, but it also included some cautions about the use of these models in the near future.
As a result, if modern schooling wants to keep up with the technological advances and ways of doing knowledge work in the “real world” outside of K-12 school buildings, traditional curricular models and pathways need to be reimagined, and fast.
For example, metacognitive reasoning skills are becoming more and more critical, while AI can be used to complete more basic tasks across a wider range of learning contexts and populations. This kind of thinking is reflected in current articulations of so-called “transportable,” “durable,” “transversal,” or “future” skills that are identified as hallmarks of postsecondary readiness and foundational components of statewide portraits of learners or graduates.
- Assessment Transformations
What do these developments mean for assessment in support of teaching and learning in the K-12 space? Assessments are instruments and, as such, they provide us evidence-based lenses on what learners are able to do under particular conditions. If we change the instrument, though, we will change our perspective and most likely learn different things about our learners, even within the same educational context.Thus, we need lenses that are best suited to the times that we live in.
More complex skill sets will need to be developed in tandem with foundational literacy skills, which some studies suggest may be successfully done through pedagogical approaches such as project-based learning and competency-based education. Often associated with terms such as “deeper learning,” these approaches commonly utilize assessment methods such as portfolios and performance tasks that are authentic, purposeful, motivating, and generative for learning. This is where AI support plays an important role again. Generative AI can accomplish tasks like this:
- Create exemplar performance tasks with instructions, resources, and learning checks
- Suggest digital resources when solving open-ended performance tasks
- Generate annotations, exemplars, and suggestions for specific revisions to work products
- Provide automated evaluation of core components of work products
- Support remote collaboration efforts by suggesting tactical next steps for problem-solving
- Suggest additional learning resources and strategies for different learners
Similarly, critical consumers must push vendors to provide innovative but trustworthy assessment solutions that leverage these modern technologies and allow all learners to demonstrate the best of what they are able to do with the appropriate support of these tools. This might lead to a form of contextual comparability of performance that is more meaningful than artificially constraining tasks for everyone in ways that seem largely decoupled from the real world, especially at higher grade levels.
Looking Ahead: Human Oversight of AI
I am not naive enough to believe that the developments I have sketched out here will all happen magically overnight. But, then again, seeing large generative multimodal models like Gemini does feel a bit like inspirational magic. We’d better learn how to be ready for the transformational pushes it is creating.
I am also fully aware that continued human oversight is necessary, given the unfortunate aspects of AI development so far. The current development teams of large corporations are increasingly diverse in ethnicity, gender identity, professional background, and interdisciplinary perspective, among other things. This diversity bodes well for insightful oversight of AI.
But even these kinds of rich, interdisciplinary teams can wear collective blinders that make them unaware of the risk and management of potential sources of bias and their associated unintended negative consequences, at least in the long run. We should continue to carefully evaluate, monitor, and improve these systems, but we should also not live in fear of them and cling to our established ways at all costs just because those risks exist.