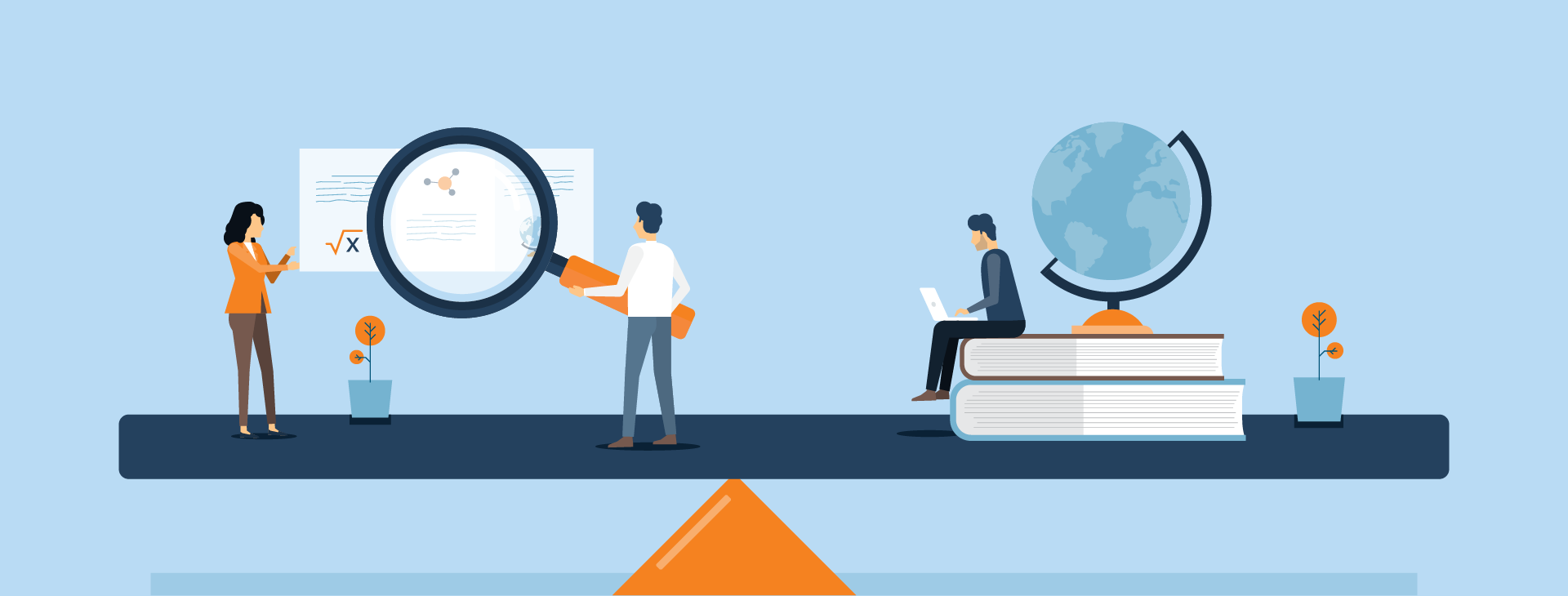
Threats to the Validity of Accountability Systems, Part 2:
Precision vs. Actionability
In our previous blog post, we described one potential threat to the validity of accountability systems: balancing the complexity and simplicity of a system’s design. In this post, we’ll talk about another potential threat: balancing precision and actionability. How precise is precise enough? And at what point does actionability—which hinges on how easily users can interpret the system’s information—become a greater priority? Unsurprisingly, the system’s guiding theory of action plays a significant role in answering these questions.
Read Parts 1 and 3 of this series on threats to accountability: Part 1 explores the balance of simplicity and complexity. Part 3 discusses how to balance formative and summative feedback.
In early measurement and research classes, it’s common to compare accuracy and precision. In this distinction, accuracy is about correctness or closeness to an accepted value, and precision is a measure’s degree of replicability. Think of a game of darts. Accuracy asks, “Did the dart hit the bullseye?” Precision asks, “Did all the darts land in the same area?” Ideally, both are true, but it’s possible to be precise without being accurate.
When evaluating accountability systems, we need a certain level of both accuracy and precision. Without sufficient precision, the system’s results are not credible. But prioritizing precision over users’ ease of interpreting its signals, or taking action in response to them, can lead to unintended negative consequences. So when might an over-reliance on precision be problematic?
Artificial Intelligence and the Risks of Precision
The proliferation of artificial intelligence and its implications for everything from manufacturing to assessment development cannot be overstated. But what happens when the data sets that AI uses to learn don’t accurately reflect the real world? The use of AI in self-driving cars offers us a recent example of the risks of over relying on precision.
The implementation of self-driving cars has gained traction quickly but required some pulling back when several incidents raised questions about their ability to account for unexpected situations. These are highly precise applications of AI using data available, yet they could not account for novel circumstances.
Precision, Actionability, and Accountability
School accountability can potentially suffer a similar fate if we over-rely on precision without considering accuracy. Both are necessary to interpret and use the system as intended. But we also need to ensure the results can be interpreted and used as intended. That is, they must be actionable.
Accountability systems for school identification are meaningless if the focus on precision is too great. There must be a focus on both precision and action, where the action helps designers focus on connections to improvement efforts or expectations for improvement. Consider the following definitions:
- Accuracy ensures appropriate schools are identified,
- Precision ensures that the collection of indicators/measures leads to reliable identification of the lowest-performing schools and subgroups, and
- Actionability ensures that the indicators/measures are easy to interpret and practically relevant to all schools, not just the lowest-performing schools
Precision and accuracy go hand-in-hand. When I refer to precision alone, it’s with the assumption that identifications are also accurate.
A hyper-focus on precision may disincentivize schools from improving. When a system encourages schools to focus too intently on whether their output is precise, school-level users risk paying less attention to what matters more: acting on information the accountability system produces.
Too much focus on precision may also send an unintended, punitive message about how lower-performing schools are ranked. Consider students who are graded based on a normative scale. Results may be accurate and precise, but motivation research tells us that lower-performing students will be (1) more likely to perform poorly in the future and (2) less motivated to engage in subsequent learning tasks.
On the other hand, focusing too intently on action without scrutinizing the accuracy and precision of the information used to produce those results undermines an accountability system. If results are not credible or trustworthy (a function of accurate and precise results), the information on which these results are based becomes useless. Therefore, accountability designers must balance the need for precision and actionability while determining a “good-enough” level of precision to maintain credibility.
Bringing Both Precision and Actionability to Design
One way to ensure that both precision and actionability are addressed in design, development, and implementation is to go back to the system’s original theory of action. What is the message that you are trying to send? How do you want to (and expect to) support schools? What schools do you want to identify? And how much precision is enough to make sound decisions?
A solid evaluation plan can help you determine quality criteria and thresholds for sufficient precision. Is the system sufficiently credible? Are users interpreting and using the data as intended? As long as there is enough evidence that the system’s results reflect a well-articulated and coherent theory of action (see Part 1 of this blog series) and that people are using the data as intended, we don’t need to chase unnecessary levels of precision.
Designers should build in just enough precision (and accuracy) to protect their system’s credibility and promote actionability by simplifying the message. Here, I offer a few practical suggestions to achieve a better balance between precision and actionability:
- Stay attuned to the signals of school quality that system designers and users want out of the system, using a theory of action as a touchstone. Check in with them and determine whether they hear the messages you want the system to convey.
- Take a critical look at the number and weights of indicators in the system. They can affect how users think they should interpret results from the accountability system. There should be an intentionality about why indicators are included in the system. Be clear and tell users why these data are important. Consider providing models of how users should engage in data review, planning efforts, and progress monitoring to maximize utility and actionability.
- Be mindful of the number of transformations in your data and your system. How many steps are needed to interpret accountability results and compare them to the original data that ground-level users are used to? Consider back-translating transformations to the original metrics so that users can monitor improvements familiarly.
Achieving a better balance between precision and actionability happens not only through design, but also by supporting the communication and interpretation of results through resources, interpretive guides, and modeled behaviors.
Read Parts 1 and 3 of this series on threats to accountability: Part 1 explores the balance of simplicity and complexity. Part 3 discusses how to balance formative and summative feedback.